Edit distance is a crucial metric in translation and localization, especially in today’s AI-driven landscape. It measures the number of changes—additions, deletions, or rearrangements—made to a given text suggestion to align it with the desired translation. This suggestion, also called a "feed," can come from various sources, including:
- Machine translation engines
- Large language models (LLMs)
- Fellow translators
The goal is to track how much effort is needed to move from the initial feed to the final, confirmed translation.
How Edit Distance is Calculated
Let’s explore how this metric works with a simple example. Suppose the feed says, “Gabriel has gone to the market.” A translator edits it to: “Gabriel went to the market.” Here’s how the calculation would look:
- Deletions: "has" and "gone" (2 edits)
- Additions: "went" (1 edit)
- Total edits: 3
- Original sentence length: 6 words
- Edit distance percentage: 3 ÷ 6 = 50%
This calculation helps quantify the translator’s effort. In some cases, changes may exceed 100% if an entirely new sentence is created, which raises the question of whether to cap the percentage or allow it to reflect the total extent of modifications.
Gabriel Fairman explains in his “Edit Distance” related video series:
"Edit distance is typically the number of edits that are done to a specific suggestion, or what people call feed that a translator receives, and the translation that the translator confirms"

Why Edit Distance Matters
In an era where translation tools rely heavily on AI, edit distance provides insight into the efficiency and quality of these tools. It offers multiple benefits:
- Assessing feed quality: Tracks how much work is needed to improve machine-generated content.
- Evaluating translator effort: Measures how much manual input is required to make translations acceptable.
- Comparing different languages or content types: Reveals patterns in how much editing specific types of content require across languages.
Together with edit time, edit distance offers a scalable way to measure the productivity and quality of translation workflows.
Challenges and Future Implications
Edit distance, though useful, has limitations. As AI-generated translations improve, fewer edits may be required. This shift raises questions:
- Is a lower edit distance always better? Not necessarily—some highly nuanced translations may still demand significant edits.
- Should percentages beyond 100% be normalized? If major rewrites are common, capping percentages at 100% could distort averages.
- What other metrics should complement edit distance? Balancing edit distance with measures of output quality and translator behavior will be key.
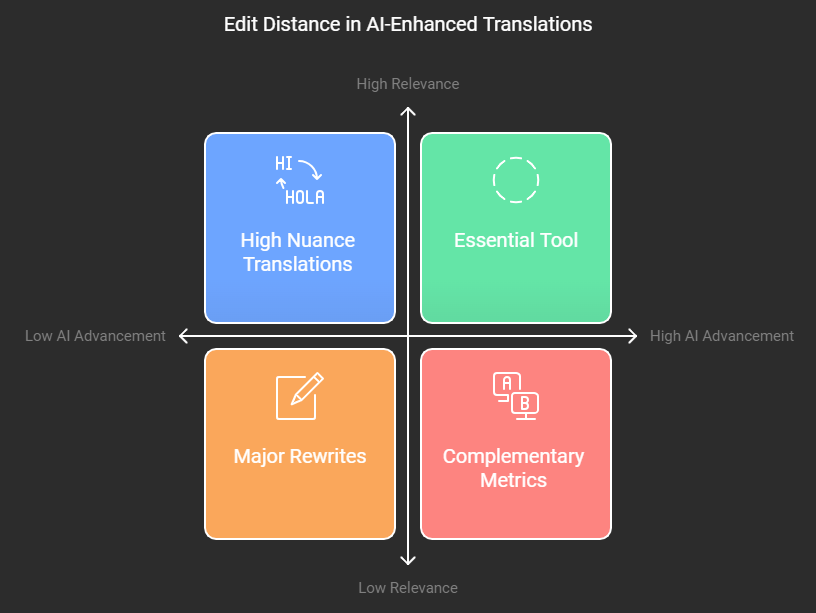
As the industry evolves, edit distance will remain an essential tool for analyzing translation efficiency, but its role will shift alongside advancements in AI. According to our CEO, Gabriel Fairman:
"As the content from large language models and machine translation engines gets better and better, we're moving into a place where we're seeing fewer and fewer edits to go from machine grade to human grade translations."
Edit distance is a fundamental metric for assessing the transformation from machine-generated suggestions to polished human translations. While it’s far from perfect, it offers a precise, scalable, and practical way to measure the translation process. As AI technologies continue to advance, monitoring edit distance will help ensure translations maintain high quality with minimal human effort.